Learn how AI is revolutionising the healthcare industry, everything including history, recent applications and its role in the present COVID crisis!
— By Sushant Varanasi, Computer Vision Researcher @Sally Robotics.

“Artificial intelligence will reach human levels by around 2029. Follow that out further to, say, 2045, we will have multiplied the intelligence, the human biological machine intelligence of our civilization a billion-fold.”―Ray Kurzweil
In this epoch of technological advancement, Artificial Intelligence has obtained appreciation in a countless number of areas. I have been pondering to what extent has AI advanced in the healthcare sector, how far we are from reaching the stage where one can consult a robot doctor or a digital doctor for medical advice. This should be without any apprehension or a situation where computers could discover the best possible drug for a disease by analysing the DNA or RNA structures of the illness-causing species.
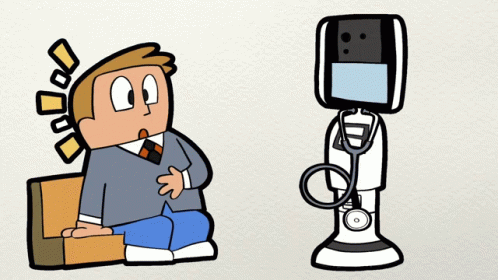
So, let us explore from the history of medical Artificial intelligence to the present-day research topics in this sector, ending with its role during this pandemic of COVID-19.
History
In this epoch of technological advancement, Artificial Intelligence has obtained appreciation in a countless number of areas. I have been pondering to what extent has AI advanced in the healthcare sector, how far we are from reaching the stage where one can consult a robot doctor or a digital doctor for medical advice. This should be without any apprehension or a situation where computers could discover the best possible drug for a disease by analyzing the DNA or RNA structures of the illness-causing species.ich would query the physician running the program via a long series of simple yes/no or textual questions.
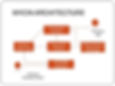
In the end, it provided a list of possible culprit bacteria ranked from high to low based on the probability of each diagnosis, its confidence in each diagnosis’ probability, the reasoning behind each diagnosis (that is, MYCIN would also list the questions and rules which led it to rank a diagnosis a particular way), and its recommended course of drug treatment.
Approaches involving fuzzy set theory, Bayesian networks and artificial neural networks have been applied to intelligent computing systems in healthcare from the 1980s and 1990s.
Current research and applications
On this day, we can find many start-ups, research topics and applications of AI in the medical sector, out of which we would inspect some of them.
Diagnostic radiology
Images from MRI machines, CT scanners, and X-rays can contain large amounts of complex data that can be difficult and time consuming for human providers to evaluate. DL algorithms can help select and extract features from medical images, identify and classify disease patterns from images and help the radiologist suggest suitable care pathways for a patient in consultation with other physicians involved in the care of the patient.
A research study by Lakhani & Sundaram (2017) used DL algorithms in the diagnoses of tuberculosis (TB) in chest X-ray images. Two different deep convolutional neural networks (DCNNs), AlexNet and GoogLeNet, were used to classify the images as having manifestations of pulmonary TB or as healthy. Both untrained and pre-trained networks on ImageNet were used, and augmentation with multiple preprocessing techniques.
Kooi et al. (2017) provided a head-to-head comparison between the state-of-the-art in the mammography CAD system, relying on a manually designed feature set and a CNN, aiming for a system that can ultimately read mammograms independently.
Creation of New Drugs
The identification of physical interactions between drug candidate compounds and target biomolecules is an important process in drug discovery. Ahmet et al. (2018) proposed a large-scale DTI (Drug target interaction) prediction system, DEEPScreen, which is composed of 704 predictive models; each one having specifically optimized hyper-parameters to accurately predict interacting small molecule ligands for a unique target protein.
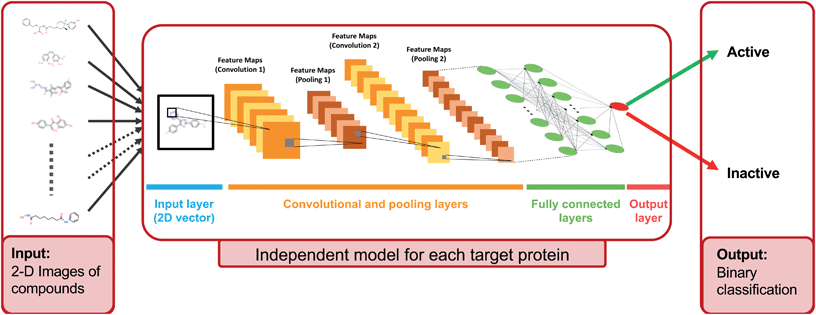
The end of January 2020 brought us the news that the first non-man made drug molecule, DSP-1181, a medicine developed by artificial intelligence may be used to treat patients with obsessive-compulsive disorder (OCD). Created through the joint venture of Sumitomo Dainippon Pharma in Japan and the UK’s start-up Exscientia (a full-stack AI drug discovery company), DSP-1181 took a single year for development, while pharmaceutical companies usually spend about five years on similar projects. It was created by using algorithms that sifted through potential compounds, checking them against a huge database of parameters.
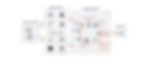
“This year was the first to have an AI-designed drug but by the end of the decade all new drugs could potentially be created by AI” ― Exscienta chief executive, Prof Andrew Hopkins
Ophthalmology
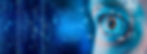
The most immediately promising computer algorithms are in the field of retinal diseases. AI-based systems are being developed to detect and evaluate even other ophthalmic conditions, including pediatric cataract, glaucoma, keratoconus, corneal ectasia, and oculoplastic reconstruction.
Some examples include diagnosing and grading cataract in pediatric patients based on an analysis of slit-lamp images (Liu et al., 2017), diagnose glaucoma based on measurement of the retinal nerve fibre layer (RNFL) thickness and visual field (VF) (Kim, Cho & Oh, 2017). Of the many other related research applications, in one of them, Dr. Schmidt-Erfurth’s group developed a fully automated segmentation algorithm for the posterior vitreous boundary. These images show the boundary in patients with (left panel) and without (right panel) vitreomacular adhesion.
based on measurement of the retinal nerve fiber layer (RNFL) thickness and visual field (VF) (Kim, Cho & Oh, 2017). Of the many other related research applications, in one of them, Dr Schmidt-Erfurth’s group developed a fully automated segmentation algorithm for the posterior vitreous boundary. These images show the boundary in patients with (left panel) and without (right panel)
Cardiology
Even cardiology has many types of research increasing the use of AI tools in its domain. Some researches have developed models to predict the risk of occurrence of cardiovascular events, categorize the patients into mutually exclusive groups to evaluate the response to resynchronization therapy cardiac, evaluate the presence of coronary disease after performing myocardial scintigraphy, a wearable device to detect arrhythmias from the information record of a single-lead electrocardiogram.
Robotic Surgery
Surgical robots are able to control the trajectory, depth, and speed of their movements with great precision, increasingly assisting microsurgical procedures to help reduce surgeon variations that could affect patient recovery. The most widely used clinical robotic surgical system includes a camera arm and mechanical arms with surgical instruments attached to them. Existing surgical robots can be grouped into four categories, each of which represents a different method for augmenting human clinicians. They are Surgeon Waldo, Programmable Automata, Assistive Guide and Motorized Laparoscopic tools.
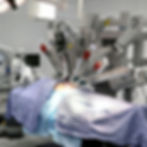
Machines like the da Vinci, TransEnterix Senhance, CMR Versius and Titan SPORT convert surgeon movements into instrument movements through computer communications between remote patient care and a physician’s console fall under “Surgeon Waldo” category. The above picture shows a Da Vinci robot. Energy delivery robots like Accuray’s CyberKnife are “Programmable Automata” that use a predefined treatment plan to calculate the positions and orientations to fire energy to focus on and destroy tumours at specific locations inside the body. Orthopaedic and dental implant robots work from a digital map of the patient, and function as an “Assistive Guide” to the human. “Motorized Laparoscopic” tools are a more modest family that bring more flexibility to straight laparoscopic sticks.
About a month ago, researchers from Google Brain, Intel AI Lab, and UC Berkeley have created Motion2Vec, an AI model capable of learning how to do tasks associated with robotic surgery — such as suturing, needle-passing, needle insertion, and tying knots — from training with surgery videos.

Motion2Vec groups similar action segments together in an embedding space in a semi-supervised manner. Pseudo-labels for the unlabelled training data is obtained by inference on an RNN that is trained iteratively for a given parameterization of the Siamese network. The learned representation is applied to surgical suturing segmentation and pose imitation in simulation and real on the da Vinci robot.
Risk prediction and alerting
KenSci, a risk prediction platform, combines big data and artificial intelligence to predict clinical, financial and operational risk by taking data from existing sources to foretell everything from who might get sick to what’s driving up a hospital’s healthcare costs.
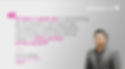
Google’s DeepMind Health AI software is being used by hospitals all over the world to help move patients from testing to treatment more efficiently. The DeepMind Health program notifies doctors when a patient’s health deteriorates and can even help in the diagnosis of ailments by combing its massive dataset for comparable symptoms. By collecting symptoms of a patient and inputting them into the DeepMind platform, doctors can diagnose quickly and more effectively.

COVID 19 (research a “need” rather than a “want”)
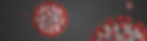
Detection via chest radiography
COVID-Net, an open-source neural network for COVID-19 detection has been created by the DawinAI team along with the researchers at the University of Waterloo’s VIP Lab in Canada. It is a convolutional neural network for COVID-19 detection via chest radiography using COVIDx, a dataset with 5941 posteroanterior chest radiography images across 2839 patient cases gathered from public sources. The following image is an example of posteroanterior chest radiography images of A) bacterial infection, B) non-COVID19 viral infection, and C) COVID-19 viral infection in COVIDx dataset.

The heavy use of a projection-expansion-projection design pattern in the COVID-Net architecture shown below can be observed, which provides enhanced representational capacity while maintaining computational efficiency.

Another proposed architecture is Deep Bayes-SqueezeNet based COVIDiagnosis-Net which classifies the three-class X-ray images labeled as Normal (no infection), Pneumonia (bacterial or none-COVID viral infection) and COVID (COVID-19 viral infection).
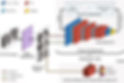
Drugs to fight COVID
Google’s DeepMind has predicted the structure of the proteins of the virus, information that could be useful in developing new drugs. atazanavir, Baricitinib and Afatinib are some drugs which have the potential for the treatment of COVID-19, found using AI. However, medical and scientific checks, trails, and controls need to be performed before these drugs will be approved.
A Mumbai-based data scientist along with his team has with the help of artificial intelligence (AI) found three molecular compounds, which they claim can be synthesised and tested further to find a novel drug to fight the Covid-19 pandemic. During their research, the team found out about a critical enzyme of the virus called 3CLpro which helps for the growth of the virus. If this enzyme gets inhibited, it can help to stop the growth of the virus. They used a process called docking to predict the inhibition.
An AI platform has also been established to identify potential old drugs with anti-coronavirus activities by using two different learning databases; one consisted of the compounds reported or proven active against SARS-CoV, SARS-CoV-2, human immunodeficiency virus, influenza virus, and the other one containing the known 3C-like protease inhibitors. Some old drugs with activities against FIP coronavirus have been identified. Further studies are underway to demonstrate their activities against SARS-CoV-2 in vitroand in vivo at clinically achievable concentrations and doses.
Other applications: Preventive Healthcare
Many tools for statistical analysis are being used for future predictions, like the risk of infection, a number of cases, deaths, identify the most vulnerable regions and people, etc.
Face Mask Detection Systems, Social Distancing Alert Systems using existing IP cameras and CCTV cameras combined with Computer Vision techniques detect people without masks and if people are at a specific distance and adhere to social distancing or not.


Conclusion
In the healthcare sector, AI is already at work increasing convenience and efficiency, reducing costs and errors, and generally making it easier for more patients to receive the health care they need.
However, the ultimate dream in healthcare is to eradicate the disease entirely. Let us see how much would AI be helping us to elevate our hopes by advancement.
“The greatest opportunity offered by AI is not reducing errors or workloads, or even curing cancer: it is the opportunity to restore the precious and time-honored connection and trust” ― Eric Topol, Deep Medicine: How Artificial Intelligence Can Make Healthcare Human Again